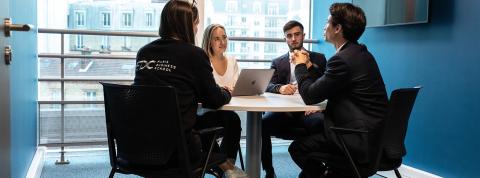
What are the most common issues and practices in ethical AI ?
Unlike humans, AI has no conscience. It does, however, face a number of ethical issues that are increasingly making the headlines. Among the most frequently discussed issues are data confidentiality, trust and environmental impact. Companies themselves have a particular interest in defining a framework and ethical standards to avoid jeopardizing their reputation. Let's take a look at the most common issues and practices in ethical AI.
Why is ethics essential in artificial intelligence ?
Artificial intelligence is transforming many aspects of our lives, from hiring decisions to disease detection. However, with this technological power comes a responsibility: to ensure that AI is used ethically and fairly. Lack of ethics can lead to bias, discrimination and negative impacts on individuals and societies.
Ethics in AI is essential for several reasons. Firstly, it ensures transparency in the decision-making processes of algorithmic systems. Understanding how an AI arrives at a conclusion is crucial to maintaining user trust and preventing abuse. Secondly, ethics aims to reduce biases in AI models, which may unwittingly reproduce or amplify existing discriminations. Finally, ethical AI minimizes unintended negative consequences, such as breaches of privacy or the dissemination of inappropriate content, while maximizing benefits for society.
Training in these issues has become essential for the growing number of professionals required to work with AI. That's why EDC Paris Business School's Master Data Science, which teaches students how to use the power of Big Data to improve business performance, includes a reflection on the ethics of AI. This program prepares students to acquire in-depth knowledge of data analysis, while tackling key themes such as Deep Learning and cybersecurity.
Algorithmic bias is one of the major challenges facing artificial intelligence. These biases, often linked to the data used to train the models, can have serious consequences:
Example 1: Discrimination in recruitment
Some AI systems used to sort applications reproduce existing biases. For example, an algorithm trained on historical data may favor male applicants if the data shows that they were predominantly hired in the past. This bias results in unfair discrimination against women or other under-represented groups.
Example 2: Racial bias in facial recognition systems
Facial recognition algorithms are often less accurate at identifying the faces of people from minority backgrounds. This can lead to serious errors, such as wrongful arrests based on erroneous recognition.
Example 3: Biased recommendations in online platforms
Algorithmic biases in recommendation engines can reinforce stereotypes or limit the diversity of content on offer. For example, an educational platform might systematically steer women towards traditionally feminine professions, such as secretaries or nurses, thus restricting their career opportunities.
Example 4: Bias in healthcare
Some AI algorithms in the medical sector, trained on unrepresentative data, can provide less accurate diagnoses or treatments for certain populations, exacerbating inequalities in access to care.
The importance of transparency and fairness in AI
In the artificial intelligence sector, transparency, justice and accountability are major issues, especially as AI is used in particularly sensitive sectors such as medical diagnostics, meteorology and transportation.
Transparency means clear communication about how algorithms work. Users and stakeholders need to be able to understand how decisions are made, whether it's approving a loan, recommending medical treatment or offering online content. This transparency is essential for building trust in AI systems, especially in contexts where confidentiality clauses restrict access to the technical details of algorithms. Even in these situations, it is possible to provide general explanations of the mechanisms used and the data analyzed, without compromising information security.
In parallel, fairness in machine learning aims to ensure that the decisions of AI systems are free from discrimination. This includes treating users fairly, regardless of their origin, gender or any other sensitive criteria. For example, in recruitment or credit-granting processes, biased models can reproduce existing social inequalities, accentuating injustices rather than mitigating them. By applying fairness machine learning techniques, it is possible to detect and correct these biases, ensuring that results are fair for all.
Alongside transparency and justice, responsibility is a central dimension of ethical artificial intelligence. It is based on the idea that the designers, developers and users of AI systems must fully assume the consequences of the decisions made by these technologies. Responsibility means clearly defining who is liable in the event of errors or malfunctions. For example, when a biased algorithm causes discrimination or a critical error, the players involved - be they the technical team, the company or its executives - must answer for the consequences.
The most common ethical practices in AI
A number of companies, including the Orange telecom group, software publisher Sage and Le Monde newspaper, have adopted a charter on the use of AI. Here are the most common practices adopted by companies wishing to control the development and use of these technologies:
Tip 1: Eliminate bias
Data science teams analyze the data used to train the models to detect and correct biases. This prevents the AI from reproducing discriminations linked to historical or cultural biases present in the datasets.
Tip 2: Be transparent in your algorithms
Companies are striving to make the decision-making processes of AI systems more comprehensible to users. This means providing explanatory reports on algorithmic mechanisms and the decisions taken, even when the models themselves are complex.
Practice 3: Developing secure systems
Ethics also include the reliability of AI solutions. Models must be tested in a variety of environments to guarantee their performance and safety, minimizing the risk of malfunctions or bad decisions. Ethical practices encourage the integration of data protection right from the design phase of AI systems. Relying on tools such as anonymization or pseudonymization, developers ensure compliance with regulations such as the RGPD.
Practice 4: Human supervision
AI systems do not have to operate entirely autonomously when making critical decisions. Ethical practices include human supervision to validate or adjust AI decisions, particularly in sensitive areas such as healthcare, finance or recruitment.
Tip 5: Reduce your environmental footprint
Artificial intelligence, because of the computing power required to process and store massive volumes of data, is an energy-intensive activity. Ethical practices include designing more energy-efficient systems, optimizing algorithms to reduce their carbon impact, and using data centers powered by renewable energies.
Ethical artificial intelligence is constantly evolving to meet technological, social and environmental challenges. In the future, we can expect advances in the management of algorithmic biases, greater transparency thanks to more advanced technologies, and better integration of environmental considerations into development models. These advances will require strengthened collaboration between researchers, companies, regulators and educators to design responsible and sustainable AI systems.