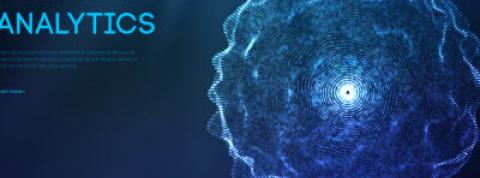
5 applications of fuzzy logic in data science
A statement isn't always true or false, and a question can elicit much more varied answers than "yes" or "no". The term "fuzzy" refers to something that is unclear or vague. Fuzzy logic in data science offers valuable flexibility for precise reasoning. If you'd like to explore this concept in depth and learn how to master many others in real-life contexts, the Master Data Science offers you a comprehensive training course to become a data science expert.
The importance of fuzzy logic for data science
Fuzzy logic, developed by mathematician Lotfi Zadeh in the 1960s, is an extension of classical logic for dealing with imprecision and uncertainty. Unlike traditional binary systems, where everything is either true or false, fuzzy logic can represent situations where values may be partially true or partially false.
This ability to model imprecise concepts makes it particularly valuable in complex and varied fields, such as data science. In data science, data is often incomplete, uncertain or ambiguous, and conventional models may be insufficient to capture these nuances. This is where fuzzy logic comes into its own, enabling us to better interpret and analyze this information with more flexible and realistic results. Whether for classifying imprecise data, segmenting customers, improving data quality, enriching data for further analysis or forecasting in uncertain environments, fuzzy logic opens the way to new approaches to data processing and analysis.
Application 1: Classification of imprecise data
In many data science projects, the data to be processed is not always clear-cut or strictly categorizable. For example, in fields such as image recognition, fraud detection or even sentiment analysis, the boundaries between different classes of data are often blurred. This is where fuzzy logic comes in, enabling data to be classified with a flexibility that better reflects reality. Classical classification is generally based on clear-cut boundaries: a piece of data belongs to one class or another. However, in situations where boundaries are ambiguous, this can lead to errors or loss of information. Fuzzy logic, on the other hand, allows data to belong partially to several classes at the same time, thanks to the concept of fuzzy membership. Each piece of data is assigned a degree of belonging to different classes, often expressed as a number between 0 and 1.
Application 2 : Recommandation systems
Recommendation systems, ubiquitous in platforms such as Netflix, Amazon or Spotify, often have to deal with subjective and ambiguous user preferences. For example, a person may like a film "a little" or "a lot", but these nuances are difficult to capture with conventional recommendation systems. Fuzzy logic offers a solution by modeling these fuzzy preferences and using them to propose more relevant recommendations.
Recommendation systems based on fuzzy logic offer several advantages:
Greater precision: by modeling preferences with degrees of certainty, recommendations become more targeted.
Improved personalization: systems can better understand the nuances of users' tastes and suggest more relevant options.
Adaptation to changing preferences: fuzzy logic enables greater flexibility in the face of evolving user preferences.
Application 3 : Forecasting in uncertain environments
In many fields, the data to be analyzed is often unpredictable and sometimes subject to complex variations. Whether it's economic, meteorological or financial data, uncertainty plays an important role, making forecasts difficult to achieve with traditional models. Fuzzy logic is proving particularly effective in integrating this uncertainty and producing more robust, realistic forecasts. In finance, stock markets are influenced by many uncertain factors, such as economic policies, social trends and even geopolitical events.
With fuzzy logic, analysts can assign degrees of certainty to these different factors to better model their impact on market fluctuations. For example, a fuzzy model might predict that there is a 70% chance that the market will rise slightly and a 30% chance that it will fall moderately, instead of giving a binary prediction.
Application 4: Customer segmentation
Customer segmentation is a key process in marketing, enabling a consumer base to be divided into homogeneous groups in order to personalize offers. However, customers don't always fall into distinct, rigid segments. They may display characteristics belonging to several segments at once. In a marketing campaign, a customer may have characteristics that make him part "young professional" and part "fitness enthusiast". Fuzzy logic enables us to deal with this reality by assigning each customer a degree of belonging to several segments, rather than forcing them to belong strictly to one.
Using fuzzy logic to segment customers has several advantages:
More realistic representation: takes into account the nuances of customer behavior and characteristics, enabling finer segmentation.
Greater personalization: thanks to more flexible segments, marketing campaigns can be better targeted, increasing their effectiveness.
Adaptability to change: fuzzy logic enables segments to be adjusted as customer data evolves, ensuring ongoing flexibility.
Application 5: Rule-based control systems
Control systems, such as those used in energy management, industrial automation or even autonomous vehicles, often have to operate in complex environments where conditions are unclear or uncertain. Fuzzy logic makes it possible to design more flexible control systems by establishing rules that incorporate this uncertainty, thus offering adapted responses to variable and imprecise situations. An energy consumption control system based on fuzzy logic could, for example, define rules such as: "if the temperature is slightly high and energy consumption is moderately high, reduce air conditioning slightly". This type of nuanced response optimizes energy efficiency while ensuring occupant comfort.
Fuzzy logic plays a key role in many areas of data science, enabling us to better manage uncertainty, model complex data and propose more nuanced and realistic solutions. If you want to master these concepts and many others, the Master Data Science at EDC Paris Business School is an ideal opportunity to acquire the skills you need to become an expert in this exciting field.